How Kai Outperforms Open AI's Chat GPT
Explore how Kai outperforms OpenAIs ChatGPT by addressing key limitations faced by large language models in the hospitality industry. From real-time data retrieval to maintaining brand voice, discover how Kai delivers accurate, on-brand responses that enhance guest experiences.
Posted by

Related reading
Why WhatsApp Is The Most Important Channel For Hotels
With over 2.7 billion users globally and more than 100 billion messages sent daily, WhatsApp has won the messaging app battle. But more importantly, how can hotels now use this platform to improve their guest satisfaction whilst also reducing the strain on their team?
Is AI The End Of Customer Service In Hospitality?
Is AI revolutionizing customer service in hospitality, or is it threatening to strip away the personal touch that makes each stay unique? Discover the potential risks and opportunities of AI in the hotel industry.
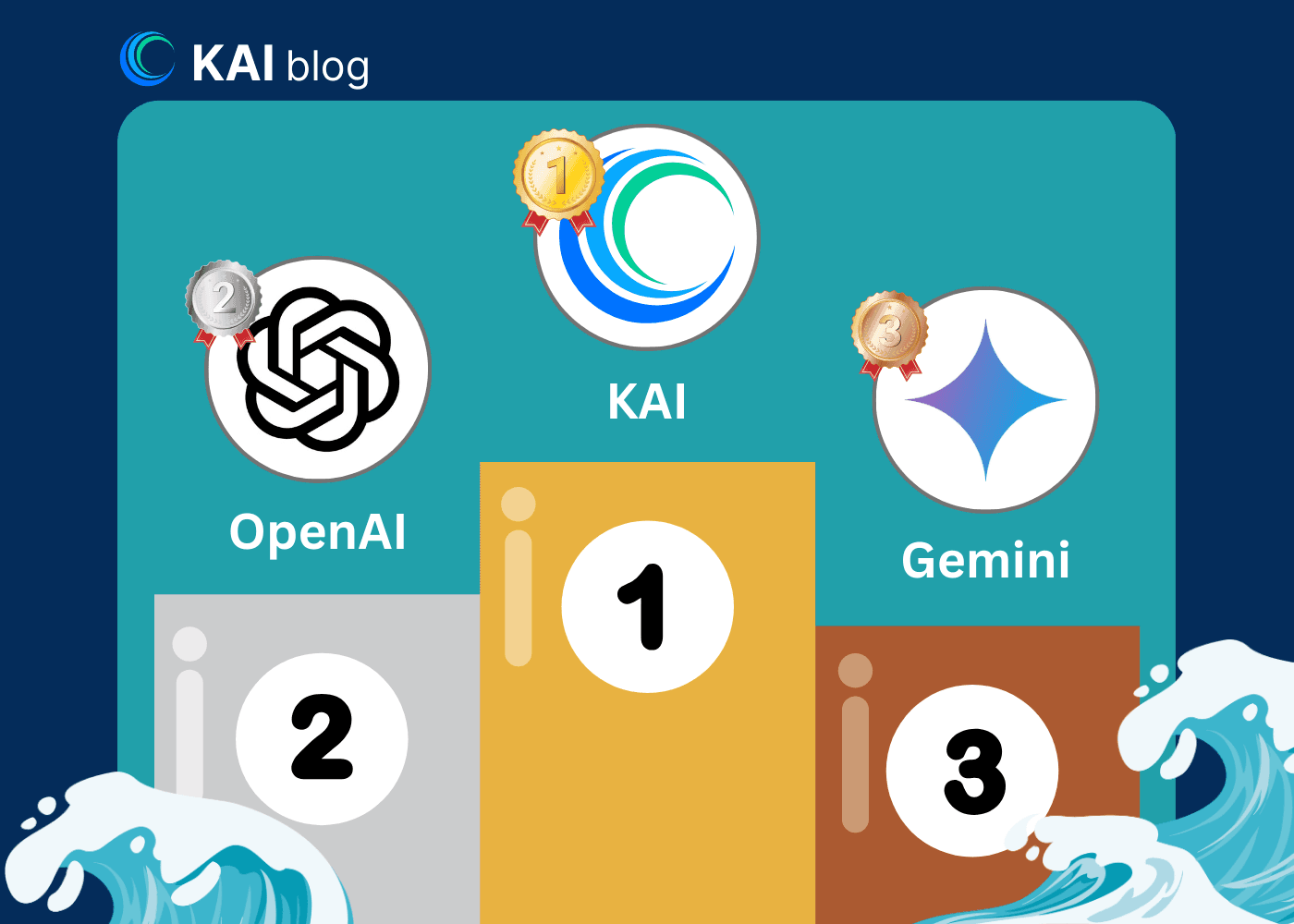
Introduction
The AI landscape is a rapidly evolving battleground, with companies racing to develop the most powerful models. Major players like OpenAI and Google have invested massive sums into creating cutting-edge AI systems such as GPT-4 and Google Gemini. OpenAI's GPT-4 alone cost an estimated $78.4 million to train, reflecting the immense computational demands and resources required to push AI capabilities forward. The competition has now progressed to demonstrating multimodal capabilities, handling text, images, audio, and even video. While these advances are often showcased in mind-blowing demos, they remain just that—demos. The real test lies in practical, user-focused applications and how these models can solve specific real-world problems. This is the metric we use to evaluate Kai, and it's how we outperform models like OpenAI's ChatGPT in delivering meaningful solutions to our customers.
The Challenges With Large Language Models
As impressive as models like GPT-4 and Gemini are, it's essential to consider the challenges of using out-of-the-box solutions to address specific problems. Particularly in the hospitality industry, hotels face challenges in communicating accurately and effectively with their guests. To support a hotel's booking team, the AI model must have detailed knowledge of the hotel, replicate the professional tone of a team member, and have access to live availability and rates. Here are some of the issues standard models face in performing these tasks:
1. Static Data
AI models like ChatGPT and Google Gemini are trained on a snapshot of data available up to a certain date, meaning they lack access to real-time information. This presents a problem for hotels, where details such as menu updates or policy changes can evolve rapidly. If a guest inquires about a restaurant's latest offerings or the current availability of services, a model relying on outdated data may deliver incorrect information, leading to customer dissatisfaction.
2. Limited API Access
These models do not inherently connect to external APIs to retrieve real-time information, such as room availability or booking statuses. While they can offer general knowledge, they struggle to answer time-sensitive queries accurately unless integrated with custom APIs. This significantly limits their practical application in the hospitality sector, where real-time data such as room availability is often critical.
3. Off-Topic Conversations
Large language models are designed to respond to a wide array of questions, which can sometimes result in off-topic discussions. In a hotel setting, a guest might inquire about hotel amenities, but the conversation could easily veer into unrelated topics, such as politics or entertainment. This detracts from the professional focus of the interaction and risks creating a less seamless and efficient guest experience.
4. Branding and Tone of Voice
Generic AI models like OpenAI's ChatGPT do not allow businesses to fully customize the interface or the tone of communication unless advanced integrations are employed. For hotels, this means losing control over the branded experience, which is crucial in hospitality, where tone, style, and professionalism are key. A chatbot interface that feels impersonal or generic can undermine the guest experience and dilute the hotel's brand identity.
5. Hallucinations
One significant risk of using AI models like GPT-4 is their tendency to "hallucinate"—to generate responses that are factually incorrect but presented confidently. In a hotel context, this could lead to the model suggesting services that do not exist or misrepresenting room features. Such misinformation can erode guest trust and damage the hotel's reputation.
Our Solution - Kai
Kai is a 24/7 AI concierge that helps booking teams respond to guests accurately in seconds, not hours. To create Kai, we leveraged the incredible progress companies like OpenAI have made, building on top of their leading models. In doing so, we addressed the challenges these models face in making them useful for specific business use cases. Below is a more detailed breakdown of how we solve these issues.
How Kai Works
Solving For Accurate Responses
Kai uses a method called Retrieval-Augmented Generation (RAG) to enhance the accuracy and reliability of its responses. Unlike static AI models that rely solely on pre-trained data, RAG allows Kai to pull relevant information from external, private data sources in real-time. This means that if a guest asks whether jet skiing is available at the resort, Kai can retrieve the latest water sports offerings from the hotel's knowledge base and present accurate information to the guest.
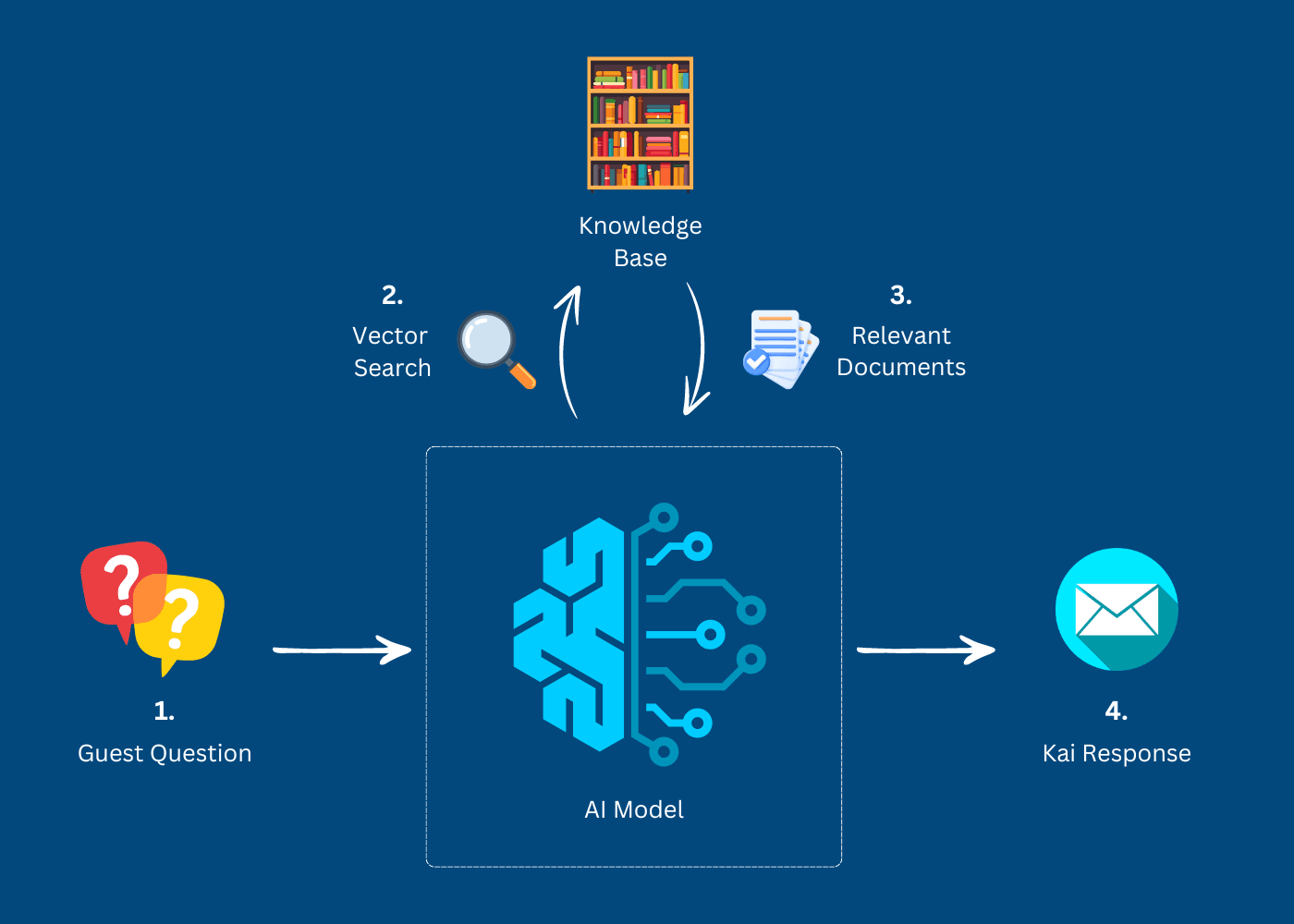
1. Diagram of Simple RAG Structure
How Does Retrieval Work?
While the concept of retrieval might seem straightforward, the actual process is more advanced than simply searching for words in a database. When a guest asks a question, such as "What types of rooms are available?", a traditional lookup might search for exact matches of the words "room" or "available." However, this method often fails to capture the broader meaning behind the question. For example, the hotel might refer to its rooms as "villas" or "beach huts," which wouldn't match the guest's search for "rooms," potentially leading to incomplete or incorrect answers.
RAG uses a process known as embeddings, where text is transformed into numerical data, creating what we call a "vector." These vectors are plotted in a multi-dimensional space where similar concepts, even if expressed with different words, are located close to one another. This allows Kai to understand that “rooms,” “villas,” and “beach huts” are semantically similar, even though they aren't exact matches in wording.
To speed up this process, all the text in the hotel's knowledge base is pre-embedded into this vector space. When a guest asks a question, that query is also converted into a vector, and the system quickly finds passages in the knowledge base that are plotted nearby. This approach, known as vector search, enables Kai to retrieve highly relevant information much faster than traditional keyword-based searches.
Moreover, by grouping similar concepts together—like various menu items or room types—Kai can pull the most contextually appropriate information without having to search through every passage in the database. This drastically improves the speed and accuracy of responses, ensuring that guests receive the correct details about their stay in near real-time. This level of precision and context-awareness is essential for meeting the high expectations of modern travellers.
Handling Off-Topic Conversations
There will always be situations where a guest's inquiry doesn't require the complex process of retrieving information from a database, and Kai is designed to handle these cases efficiently. For example, a guest might send a simple greeting like "Hello!" or "Anyone there?" In these instances, there is no need to search through the knowledge base, as the response should be straightforward and conversational.
To manage these types of questions, Kai uses a technique called Guardrails, which helps the system recognize when a response doesn't require deep retrieval. Guardrails act as quick filters, categorizing the guest's message based on its content. For instance, common phrases like greetings or even profanity can be quickly identified and routed to a specific set of responses. If a guest says something inappropriate, the AI can respond professionally while keeping the conversation aligned with the hotel's values.
This filtering process ensures that Kai can instantly handle general comments, off-topic questions, or even inappropriate language without the need for unnecessary computation. It also maintains the professionalism expected in hospitality settings, ensuring a smooth and respectful interaction, no matter what type of input the guest provides.
Increasing the Functionality of the Solution
The next step involves running the user input through a decision-making LLM. This model has access to various functions, one of which is the RAG method to retrieve relevant context. Another function might access room availability via an API, while another could involve sending an email to the booking team if the issue requires human attention. The user input, along with the context, is passed through this decision-making LLM to determine the most appropriate action. This approach also acts as a secondary defense mechanism, ensuring that if none of the available functions are suitable, the query is handled appropriately.
Generating an Accurate, On-Brand Response
Once the RAG or other function is completed, the next step is to generate a response using the collected information and user input. The first challenge is ensuring the tone of voice aligns with the hotel's brand. One way to achieve this is through the system prompt, which provides instructions to the AI model in plain language. By testing different prompts, you can consistently guide the AI's behavior and role. For more refined control, the model can be fine-tuned. This involves taking a pre-trained model, such as OpenAI's GPT-4, and making small adjustments to ensure the output matches the desired tone and style.
To ensure accuracy, the AI can be prompted to only use the provided context and avoid generating false information (also known as hallucinations). The risk of hallucinations can be further reduced by running the response through another LLM, which reviews the input and output to check for errors. This self-review step significantly improves the accuracy of responses to guests.
Summary
Large language models have made incredible advancements in recent years, but there are still gaps between their capabilities and how businesses can utilize them to solve specific real-world problems. At Kai, we are solving this by building solutions specifically for hotel booking teams that leverage the power of AI. This supports teams in communicating accurately and efficiently with guests, ultimately leading to more bookings and higher revenue. It's essential to consider the limitations of large language models, which are built to perform a wide range of tasks but need to be tailored to address specific business challenges.
If you'd like to learn more about how Kai can support your hotel, please contact us at contact@hellokai.ai.